The Faculty of Science and Technology of the University of Latvia (UL) has become an important participant in the European Research Council (ERC) Synergy Project "EUROpest". This six-year project combines interdisciplinary methods to study historical pandemics and their impact in Europe. The Baltic region's paleoecological research, led by Professor Normunds Stivriņš from the Department of Geography at UL’s Faculty of Science and Technology, will provide new insights into human-nature interactions in the context of historical pandemics, helping to better understand the course and consequences of pandemics.
In the prestigious European Research Council’s (ERC) Synergy Grant "EUROpest", Professor Normunds Stivriņš will lead studies on the paleoecology of the Baltic region and the dynamics of early disease outbreaks.
The "EUROpest" project, led by Professor Adam Izdebski from Warsaw University, combines historical, natural science, and machine learning methods to understand how different social and ecological factors in pre-industrial Europe (1300–1800) influenced disease spread and its consequences. The research focuses on the question: why did one wave of a pandemic cause severe consequences in one region, while it was nearly unnoticed elsewhere? This six-year initiative will provide new insights into why studying only the pathogen is insufficient to predict the course and outcomes of pandemics.
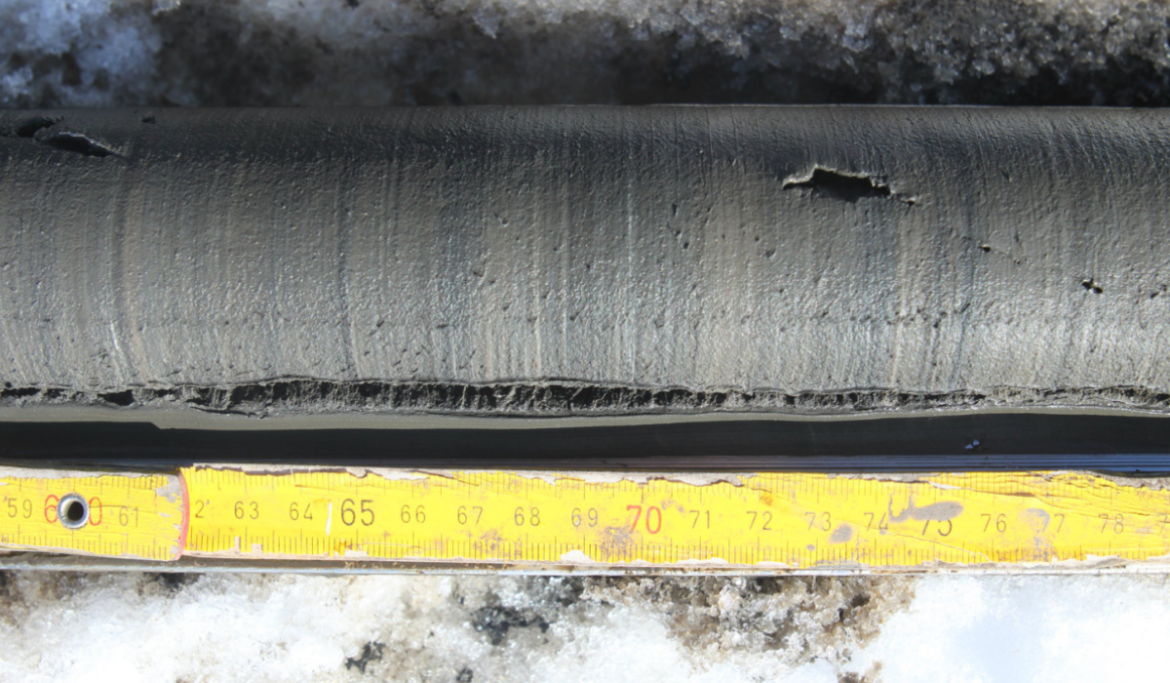
UL’s Faculty of Science and Technology will serve as the central research platform for the Baltic region, analyzing human-nature interactions using evidence from lake and bog sediments, as well as cultural layers related to early pandemics. Under the guidance of Professor Stivriņš, a series of studies will be conducted, based on archaeological and historical data, as well as climate and ecological change analysis. These studies will help clarify how Baltic societies dealt with epidemics and how human and natural factors influenced the pattern of disease spread.
"This Synergy Grant demonstrates UL’s international research level and our contribution to significant global initiatives," says Professor Stivriņš.
The project’s results will help understand how factors such as climate, social structure, economic conditions, and cultural practices influenced the course and consequences of pandemics. The knowledge gained could facilitate the development of theoretical models to predict and manage future pandemics.
About the "EUROpest" Project:
"EUROpest" is the result of interdisciplinary collaboration, aiming to reflect the complex ways in which socio-ecological systems shape epidemics. It is based on resilience-participant and network theories to develop an eco-bio-social paradigm, incorporating a multi-causal understanding of disease transmission and the impact of diseases on human societies and ecosystems. For its laboratory, "EUROpest" uses the well-documented and diverse European world of the late Middle Ages and early modern period, studying its interconnected climatic, cultural, demographic, economic, ecological, and pathogenic disease histories. Regarding the latter, "EUROpest" assumes that malaria, smallpox, tuberculosis, and intestinal diseases were the major disease components during the spread of the most devastating disease—plague (Y. pestis).
"EUROpest" will conduct regional case studies from Spain to Lithuania and from Greece to England. The research regions were selected based on the availability of written, scientific, and archaeological data. The context of plague outbreaks identified in these regions will be examined holistically to understand how the environment contributed to the outbreaks, shaped them, and their short- and medium-term impacts. "EUROpest" combines archival source analysis with archaeology, archaeogenetics, paleoecology, and paleoclimatology, and case study research will be subjected to a new, human-supervised machine learning method to determine the causal role of influencing factors.